The first Macau Forum on Emotional Artificial Intelligence, organized by the Institute of Collaborative Innovation at the University of Macau, will be held on 16 April 2025 in Macau. This forum will explore the latest advancements in affective computing and AI-driven emotional awareness.
Discover how internationally leading experts cross interdisciplinary divides—from computer science, robotics, and cognitive neuroscience to linguistics, communication, and psychology—to investigate the next frontier in artificial intelligence: emotion. How do cutting-edge technologies enable the detection of human emotions from language and text, nonverbal and implicit sources, and signals from the brain itself? How can we use such information to create emotionally capable intelligent robots and virtual agents?
With this forum, we aim to discuss the possibilities and challenges—practically, philosophically, and ethically—of creating a common ground for advancing AI towards emotional awareness and infusing human-AI interaction with feeling.
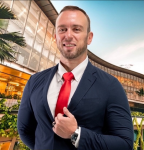
Prof. Erik CAMBRIA
Nanyang Technological University
Erik Cambria is a Professor at Nanyang Technological University, where he also holds the appointment of Provost Chair in Computer Science and Engineering, and Founder of several AI companies, such as SenticNet (https://business.sentic.net), offering B2B sentiment analysis services, and finaXai (https://finaxai.com), providing fully explainable financial insights. Prior to moving to Singapore, he worked at Microsoft Research Asia (Beijing) and HP Labs India (Bangalore), after earning his PhD through a joint program between the University of Stirling (UK) and MIT Media Lab (USA). Today, his research focuses on neurosymbolic AI for interpretable, trustworthy, and explainable affective computing in domains like social media monitoring, financial forecasting, and AI for social good. He is ranked in Clarivate’s Highly Cited Researchers List of World’s Top 1% Scientists, is recipient of many awards, e.g., IEEE Outstanding Early Career, was listed among the AI’s 10 to Watch, and was featured in Forbes as one of the 5 People Building Our AI Future. He is an IEEE Fellow, Associate Editor of various top-tier AI journals, e.g., Information Fusion and IEEE Transactions on Affective Computing, and is involved in several international conferences as keynote speaker, program chair and committee member.
7 Pillars for the Future of AI
In recent years, AI research has showcased tremendous potential to impact positively humanity and society. Although AI frequently outperforms humans in tasks related to classification and pattern recognition, it continues to face challenges when dealing with complex tasks such as intuitive decision-making, sense disambiguation, sarcasm detection, and narrative understanding, as these require advanced kinds of reasoning, e.g., commonsense reasoning and causal reasoning, which have not been emulated satisfactorily yet. The Seven Pillars for the future of AI (https://sentic.net/7-pillars-for-the-future-of-ai.pdf) address these shortcomings and pave the way for more efficient, scalable, safe and trustworthy AI systems.
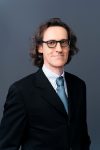
Prof. Johan F. HOORN
The Hong Kong Polytechnic University
Hoorn holds two PhD degrees: in Computer Science and in General Literature, now working in the field of inferential AI, epistemics, and theory of creativity. Hoorn’s research intersects with areas such as affective computing, social robotics, and the development of computational models that simulate human-like behavior, contributing to the understanding of how personality and emotion can be integrated into the design of robots and virtual agents. His work in the area of emotional ambiguity and vagueness in affective decision making is oriented at quantum probability and computation. His latest work is dedicated to the quantum epistemics of the observer effect.
A fuzzy model of human affect with quantum extensions, regulating the LLM while explaining its emotional decisions
In the UGC-funded project Social Robots with Embedded Large Language Models Releasing Stress among the Hong Kong Population, we aim to develop social robots, AI, and technical infrastructure that can provide customised mental care and support, enhancing the well-being of those underserved by an overburdened healthcare system. We do basic research into fuzzy logics and quantum probability of affective decision making, reality perception, and the observer effect and apply it to a robot’s perspective taking and capability to adapt to human indecisiveness, emotional ambiguity, and vagueness. In this presentation, we delve into the Silicon Coppélia model, generating affective responses, thereby overlaying the LLM to keep disadvantageous responses in check.
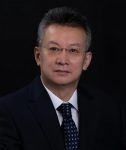
Prof. Bin HU
Beijing Institute of Technology
Bin Hu is a (Full) Professor and the Dean of the School of Medical Technology at Beijing Institute of Technology, China. He is a National Distinguished Expert, Chief Scientist of 973 as well as National Advanced Worker in 2020. He is a Fellow of IEEE/IET/AAIA and IET Fellow Assessor & Fellowship Advisor. He serves as the Editor-in-Chief for the IEEE Transactions on Computational Social Systems and an Associate Editor for IEEE Transactions on Affective Computing. He is one of Clarivate Highly Cited Researchers, World’s Top 2% Scientists and 0.05% Highly Ranked Scholar from ScholarGPS.
Computational Psychophysiology and Mental Health
In recent years, mental health issues have become increasingly prominent all of the world. According to the report from the World Health Organization, approximately 970 million people suffer from mental disorders, accounting for 13% of the global population. Currently, the diagnosis of mental illnesses primarily relies on physician interviews and Brief Psychiatric Rating Scale (BPRS), lacking objective and quantifiable diagnostic indicators. Besides, the common treatment of mental disorders is pharmacotherapy, which is often associated with significant side effects. The rapid advancement of cutting-edge artificial intelligence and big data technologies offers new opportunities for the diagnosis and treatment of mental disorders. These technologies are shifting the approach to data driven screening and treatment, offering more precise, personalized, and effective solutions. This report will introduce the opportunities and challenges in the field of medical electronics and computational methodologies for the diagnosis and treatment of mental disorders.
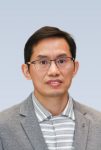
Prof. Minlie HUANG
Tsinghua University
Dr. Minlie Huang, professor of Tsinghua University, the deputy director of the Foundation Model Center of Tsinghua University. He was supported by National Distinguished Young Scholar project. He won several awards in Chinese AI and information processing societies, including Wuwenjun Technical Advancement Award and Qianweichang Technical Innovation Award. His research fields include large-scale language models, language generation, AI safety and alignment, social intelligence, etc. He authored a Chinese book “Modern Natural Language Generation”. He published more than 200 papers in premier conferences and journals (ICML, ICLR, Neurips, ACL, EMNLP etc.), with more than 25,000 citations, and was selected as Elsevier China’s Highly Cited Scholars since 2022 and the AI 2000 list of the world’s most influential AI scholars since 2020; He has won several best papers or nominations at major international conferences (IJCAI, ACL, SIGDIAL, NLPCC, etc.). He led the development of several pretrained models including Emohaa and CharacterGLM. He serves as associate editors for TNNLS, TACL, CL, and TBD, and has served as the senior area chair of ACL/EMNLP/IJCAI/AAAI for more than 10 times. His homepage is located at http://coai.cs.tsinghua.edu.cn/hml/.
Social Intelligence with LLMs: on Emotion, Mind and Cognition
Today’s LLM is designed as a machine tool to facilitate the efficiency, productivity, & creativity of human works.
However, social intelligence, which is a significant feature of human intelligence, has been largely neglected in current research.
Future AGI must have not only machine intelligence but also social intelligence. In this talk, the speaker will talk about how to embrace social intelligence with LLMs, for emotion understanding, emotional support, modeling cognition and theory of mind, and applications for mental health.
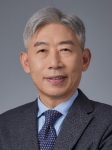
Prof. Bao-Liang LU
Shanghai Jiao Tong University
Bao-Liang Lu received the Ph. D. degree in electrical engineering from Kyoto University, Kyoto, Japan, in 1994. Since August 2002, he has been a full professor at the Department of Computer Science and Engineering, Shanghai Jiao Tong University, China. He is the directors of the Center for Brain-Like Computing and Machine Intelligence and the Key Laboratory of Shanghai Education Commission for Intelligent Interaction and Cognitive Engineering. He received 2018 IEEE Transactions on Autonomous Mental Development Outstanding Paper Award, 2020 Wu Wenjun Artificial Intelligence Natural Science First Prize, 2021 Best of IEEE Transactions on Affective Computing Paper Collection, 2022 the ACM Multimedia Top Paper Award, and 2022 Asia Pacific Neural Network Society Outstanding Achievement Award. He is Associate Editors of IEEE Transactions on Affective Computing and Journal of Neural Engineering and the IEEE Fellow. His research interests include deep learning, large EEG model, emotion artificial intelligence, and affective brain-computer interface.
Affective Brain-Computer Interface: Characteristics, Algorithms and Applications
Affective brain-computer interface is a type of brain-computer interface that decodes and regulates people’s emotions. Compared with motor brain-computer interfaces, the affective brain-computer interface has two important characteristics, one is that it needs a variety of emotion-induced paradigms and materials, and the other is that it can use EEG signals and other physiological and non-physiological signals. In this talk, I will introduce some basic characteristics of affective brain-computer interfaces, several typical deep learning algorithms for building emotion recognition models, and large EEG models recently developed, and finally I will introduce the application of multimodal affective brain-computer interfaces in the objective assessment of depression.
Invited Speakers
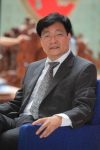
Prof. Cheng-Zhong XU
University of Macau
Dr. Cheng-Zhong Xu, IEEE Fellow, is the Dean of the Faculty of Science and Technology, University of Macau, Macao SAR, China and a Chair Professor of Computer Science of UM. He was also a Chief Scientist of key project on “Internet of Things for Smart City” of Ministry of Science and Technology of China and key project on “Intelligent Driving” of Macau SAR. He was a Chief Scientist of Shenzhen Institutes of Advanced Technology (SIAT) of Chinese Academy of Sciences. Prior to these, he was in the faculty of Wayne State University, USA. Dr. Xu’s research interest is mainly in the areas of parallel and distributed computing, cloud and edge for AI, autonomous driving and intelligent transportation. He published over 500 papers and received 21K+ citations. Notably, his work was cited by more than 300 international patents, including 225 USA patents. He received his B.S. and M.S. degrees in Computer Science from Nanjing University and Ph.D. from the University of Hong Kong in 1993.
Overview of Macao Emotional AI
This talk will introduce a recently launched project MEA: Macao Emotional AI. AI is making breakthroughs recent years, imposing profound impacts on every aspect of life and society. Recent advances are largely with AI agent’s knowledge and reasoning capability but with limited success on emotions. MoEAI project aims to empower AI agent’s capability of regulating human emotions in latest machine learning technologies. It is conducted in three aspects: multimodal emotion recognition, online validation of quantified emotions, and real-time emotion regulation. Two demo applications are under development: elderly caring and autonomous driving.
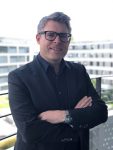
Prof. Christian MONTAG
University of Macau
Dr. Christian Montag works at the intersection of psychology, computer science, neuroscience and behavioral economics. Using a multimethodological approach he studies how artificial intelligence is impacting upon individuals and societies.
Before his position at University of Macau he served for more than ten years as Full-Professor (W3) of Molecular Psychology at Ulm University in Ulm, Germany. He was also Agreement-Professor at University of Electronic Science and Technology of China in Chengdu, China (six years) and since autumn 2023 he is Adjunct-Professor at Hamad Bin Khalifa University in Doha, Qatar.
He is author of numerous papers and books.
More information: https://www.researchgate.net/profile/Christian-Montag
Basic emotion theorists vs. social constructivists in the age of AI
Much affective computing relies on the idea of basic emotions, hence that distinct emotional expressions in faces (and underlying brain systems) are conserved across different ethnic groups.
This idea has been challenged by the social constructivists in the field of emotion research stressing that social experiences shape the way we experience and label emotions.
Both the basic emotion theorists’ view as depicted via Ekman’s psychological and Panksepp’s neuroscientific works will be contrasted with Feldman-Barrett’s social constructivist view. It will be discussed what this means for the scientific approach of studying emotions via AI methods.
After this more theoretical discussion, data will be presented showing that – despite this dispute – artificial intelligence can help to meaningfully detect emotional expressions in portrait pictures uploaded to social media and to show how these emotional expressions are linked to personality.
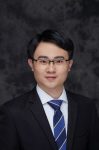
Prof. Qi LI
Institute of Automation,
Chinese Academy of Sciences
Qi Li is an Associate Researcher at the Institute of Automation, CAS, and a member of the CAS Youth Innovation Promotion Association. He has published 20 papers in CCF Tier-A journals and conferences as the first or corresponding author. His work AnyFace was a CVPR Best Paper Candidate and featured in TPAMI’s Best of CVPR. He has led multiple national projects, served as ICLR 2025 Area Chair, and held key roles in CCBR. His research has earned awards like the CSIG Technical Invention Award (2022, Second Prize) and the Industry-University-Research Innovation Award (2023, Second Prize).
Multimodal Physiological and Psychological Intelligent Perception Under Human Micromotion Patterns
Affective and psychological perception are pivotal in human-machine interaction and essential domains within artificial intelligence. Existing physiological signal-based affective and psychological datasets primarily rely on contact-based sensors, potentially introducing extraneous affectives during the measurement process. Consequently, creating accurate non-contact affective and psychological perception datasets is crucial for overcoming these limitations and advancing affective intelligence. In this talk, we introduce the Remote
Multimodal Affective and Psychological (ReMAP) dataset, for the first time, apply head micro-tremor (HMT) signals for affective and psychological perception. ReMAP features 68 participants and comprises two sub-datasets. The stimuli videos utilized for affective perception undergo rigorous screening to ensure the efficacy and universality of affective elicitation. Additionally, we propose a novel remote affective and psychological perception framework, leveraging multimodal complementarity and interrelationships to
enhance affective and psychological perception capabilities.
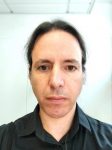
Prof. Michiel SPAPE
University of Macau
Michiel Spapé is a cognitive psychologist with interests in consciousness, intention, and emotion. While working on his PhD (Leiden University, Netherlands), he realized the potential of new interaction technologies to inform our thinking about ancient questions related to these subjects, especially when used in combination with neuroimaging. Continuing down this road, he did several projects in the UK and Finland, studying how touch and emotion relate using haptic devices in VR and EEG. Now, based in Macau, he continues studies consciousness and emotional AI with neuroadaptive brain-computer interfacing, generative AI, and XR.
Seeing versus feeling emotions: Multimodal detection of emotional imagery and prediction across tasks
Affective computing involves a promise that by detecting and learning to predict emotions, we may make artificial systems affectively intelligent, thereby optimizing interaction and wellbeing. Although recent years have shown rapid improvement of classification of emotions from biosignals obtained while viewing emotional images, I argue this severely underestimates the challenge of detecting actual feelings rather than visual differences in pictures, which means models cannot be applied in the field. Indeed, a better paradigm for detecting emotions is in the complete absence of confounding perception, for which reason I created the first multimodal, affective imagery dataset. Recordings of EEG, facial EMG, EDA, ECG, accelerometer data, and EOG were made for 39 users. These engaged in tasks measuring affective model cross-task performance. In the first task, participants were requested to imagine having emotions differentiated in emotional arousal and valence, while in the second, participants were asked to imagine being in situations that were chosen for their likelihood of eliciting similar emotions. To verify emotional detection was unaffected by a secondary task, users were furthermore requested to keep track of time. Despite the richness of the data, and high within-task emotion detection accuracy, we found very limited ability of cross-task capability, despite the two tasks being nearly identical in nature. To prove this is not related to a failure in machine learning, I present the open dataset as a challenge to the affective computing community. If others can demonstrate emotion detection without relying on confounded measures or superficial pattern detection, I will withdraw my claim that how the mind and brain represent emotions creates fundamental constraints to the more superficial devices applied for emotion detection such as EEG. In conclusion, I argue that affective computing should concentrate on whether we feel emotional, not whether a picture looks emotional.
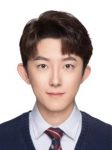
Prof. Zhenning LI
University of Macau
Zhenning Li is currently an Assistant Professor at the State Key Laboratory of Internet of Things for Smart City and the Department of Computer and Information Science, University of Macau. His research focuses on connected autonomous vehicles and Big Data applications in urban transportation systems. He has published over 60 papers in leading journals and conferences. His contributions have earned him multiple accolades, such as the Macau Science and Technology Award, the TRB Best Young Researcher Award, the Chinese Government Award for Outstanding Self-Financed Students Abroad, and the CICTP Best Paper Award. He also served as editors for various journals, such as IEEE T-TIS, Journal of Safety Research, Results in Engineering.
Human-like autonomous driving planning in mixed autonomy environment
In mixed autonomy environments, autonomous vehicles (AVs) must interact seamlessly with human drivers, requiring planning strategies that balance safety, efficiency, and social compliance. Traditional methods often lack human-like adaptability, leading to rigid or unnatural behavior. This talk explores a planning framework that integrates trajectory learning and human behavioral modeling to enable AVs to drive more naturally. By leveraging multimodal perception and social-aware decision-making, our approach improves AV adaptability, reducing intervention rates and enhancing traffic flow. The goal is to develop AVs that interact smoothly with human drivers, fostering safer and more efficient road integration.
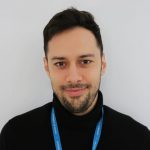
Prof. Steven MORAD
University of Macau
Steven’s focus is on deep reinforcement learning and how we can use long-term memory to improve the reasoning capabilities of artificial agents. He is an assistant professor with joint appointments in the department of Computer and Information Science, as well as the Centre for AI Research within the Institute of Collaborate Innovation. Steven completed his PhD in computer science at Cambridge, his master’s in aerospace engineering at the University of Arizona, and his bachelor’s in computer science at the University of California, Santa Cruz.
Reinforcement Learning with Long-Term Memory
Agents trained with deep reinforcement learning can benefit from long-term memory, improving reasoning capabilities just as it does in humans. In this talk, I will give an overview of my research on combining learn term memory with deep reinforcement learning, and some recent and surprising findings suggesting the emergence of fear in our memory-endowed agents.
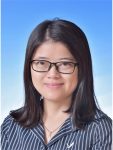
Prof. Haiyan WU
University of Macau
Professor Haiyan Wu is the Principal Investigator of ANDlab at the CCBS of University of Macau (https://andlab-um.com/). Before joining the University of Macau, Professor Wu obtained her Ph.D. from Beijing Normal University and served as a researcher at the Institute of Psychology, Chinese Academy of Sciences. Her research focuses on developing an interdisciplinary technological framework that integrates artificial intelligence, brain imaging, computational models, intracranial and extracranial neural signals, neural modulation, virtual reality, and big data to explore social neuroscience. Her work primarily centers on the interaction between emotion and decision-making in the brain. She has more than 50 publications, and her publications have been cited over 2200 times. She is a member of the Young Editorial Board of Communication Psychology, and Neuroscience Bulletin. In 2023, she was recognized as one of the “Top 30 Young Innovators in Brain Science and Artificial Intelligence.”
Unraveling Emotional Intelligence in Large Language Models: Implications for Psychology
This presentation explores the intriguing prospect of large language models (LLMs) acquiring emotional intelligence and their potential applications within the field of psychology. We will initiate the discussion by defining human emotional intelligence and tracing the historical development of LLMs. Despite their impressive capabilities in natural language processing, such as text comprehension and generation, current research has largely overlooked the crucial facets of emotion identification, understanding, and expression in these models.
To address this gap, we advocate for the implementation of rigorous assessments that gauge the emotional intelligence of LLMs, drawing on psychological constructs and methodologies. We review existing research aimed at evaluating the emotional intelligence of these models and make a case for the integration of LLMs into psychological research as a new area, particularly concerning their emotional and moral implications. Furthermore, this talk will delve into the significant role that LLMs can play as instruments for advancing our understanding of emotional psychology in the contemporary landscape, viewed through the lens of emotional cognitive science. By fostering a dialogue between AI and psychology, we aim to illuminate potential pathways for future research and applications that harness the emotional capabilities of LLMs.
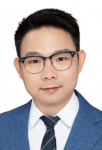
Prof. Junwen ZHONG
University of Macau
Professor Zhong Junwen is an Assistant Professor at the Department of Electromechanical Engineering, Faculty of Science and Technology, University of Macau. He has been engaged in fundamental and applied research in the field of flexible electromechanical sensors/actuators for a long time and has achieved a number of innovative research results. He has published more than 60 papers in renowned journals such as Science Robotics, Nature Communications, IEEE Transactions on Robotics (T-RO), and Advanced Materials as the first/corresponding author. The total number of citations for his published papers has reached over 8860, with an H-index of 41.
Piezoelectret-based flexible electromechanical transducers for emotional AI
A fully interconnected and intelligent living environment has been a grand challenge for future smart society and it is critically essential to develop the interactive sensing and actuating systems to bridge the human-machine interfaces. Here, flexible electromechanical transducers based on high-performance piezoelectret polymers are fabricated to selectively perform either the actuating or sensing function. As sensors, both low pressure detection limit for sensing resolution and excellent stability have been achieved, with typical application in monitoring human or machine motions. As actuators, mechanical vibrations with large output force and displacement have been produced for providing rich haptics feedback to human skin or expressing emotions. These flexible electromechanical transducers are key hardware foundations for constructing emotional AI.
參會鏈接點此或掃描下方二維碼 (免費注冊)
Please click HERE or scan the below QR Code for registration (Free for registration)
澳門特別行政區,澳門大學N21-G013室
N21-G013 University of Macau, Avenida da Universidade, Taipa, Macau SAR
Click here for our CAMPUS MAP
Prof. Chengzhong XU, University of Macau
Prof. Michiel SPAPE, University of Macau
Prof. Haiyan WU, University of Macau
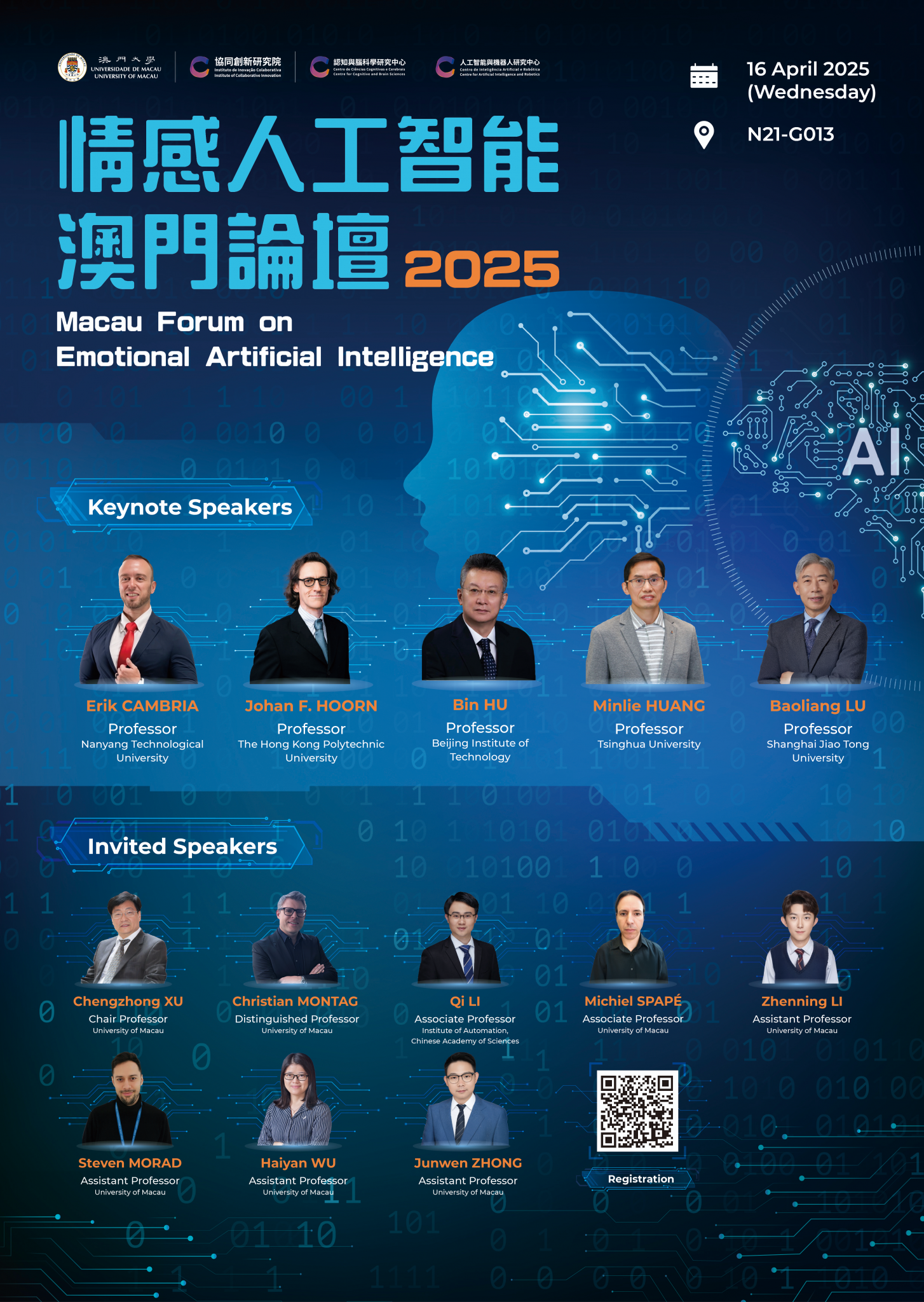