Professor Rihui Li’s research team from the Center for Cognitive and Brain Sciences at the University of Macau published a methodological study titled “Disentangling the impact of motion artifact correction algorithms on functional near-infrared spectroscopy-based brain network analysis.” in Neurophotonics (IF: 4.8, JCR Q1), the flagship journal of the Society for functional near-infrared spectroscopy (SfNIRS).
Functional NIRS (fNIRS), known for its high ecological validity, is extensively used in brain functional network research. Motion artifact (MA) is a major source of noise induced during fNIRS data recording, which can be removed by various MA correction methods. However, it remains unclear how various MA correction methods altered fNIRS-based functional connectivity (FC) and topology analyses. In this study, the team examined the effects of various MA correction algorithms on brain FC analysis using fNIRS.
Using both simulated and real-world datasets, the team systematically evaluated several MA correction algorithms, including principal component analysis (PCA), spline interpolation, correlation-based signal improvement (CBSI), Kalman filtering, Wavelet filtering, and temporal derivative distribution repair (TDDR). The mean FC of predefined networks, receiver operating characteristic (ROC) curves, and graph theory metrics were utilized to quantify the performance of each algorithm.
The results revealed that while most algorithms performed similarly, TDDR and wavelet filtering stood out as the most effective methods. These two algorithms demonstrated superior denoising capabilities, as evidenced by the best ROC results and enhanced ability to recover the original FC and topological patterns.
The findings from this study illuminate the diverse impacts of MA correction algorithms on brain FC analysis, providing a crucial reference for selecting appropriate methods in future FC research. Based on their results, the team recommends TDDR or Wavelet filtering for minimizing the influence of MA correction on brain network analyses.
This research was conducted by PhD student Shuo Guan from the Center for Cognitive and Brain Sciences, under the guidance of Professor Rihui Li, who served as the corresponding author. The project is supported by the National Natural Science Foundation of China (Reference No. 82301743), the Science and Technology Development Fund of the Macao SAR (Grant No. 0010/2023/ITP1), and the University of Macau (Grant No. SRG2023-00015-ICI). The full text can be accessed here: https://doi.org/10.1117/1.nph.11.4.045006.
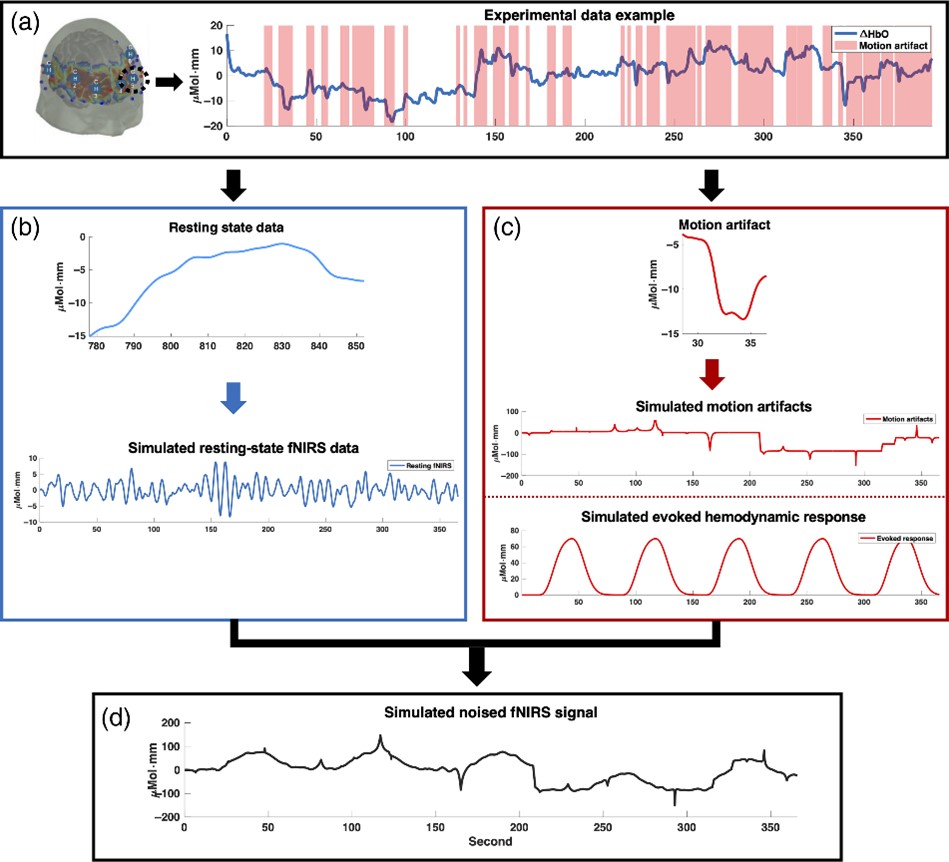
Fig.1. Pipeline of the fNIRS data simulation.
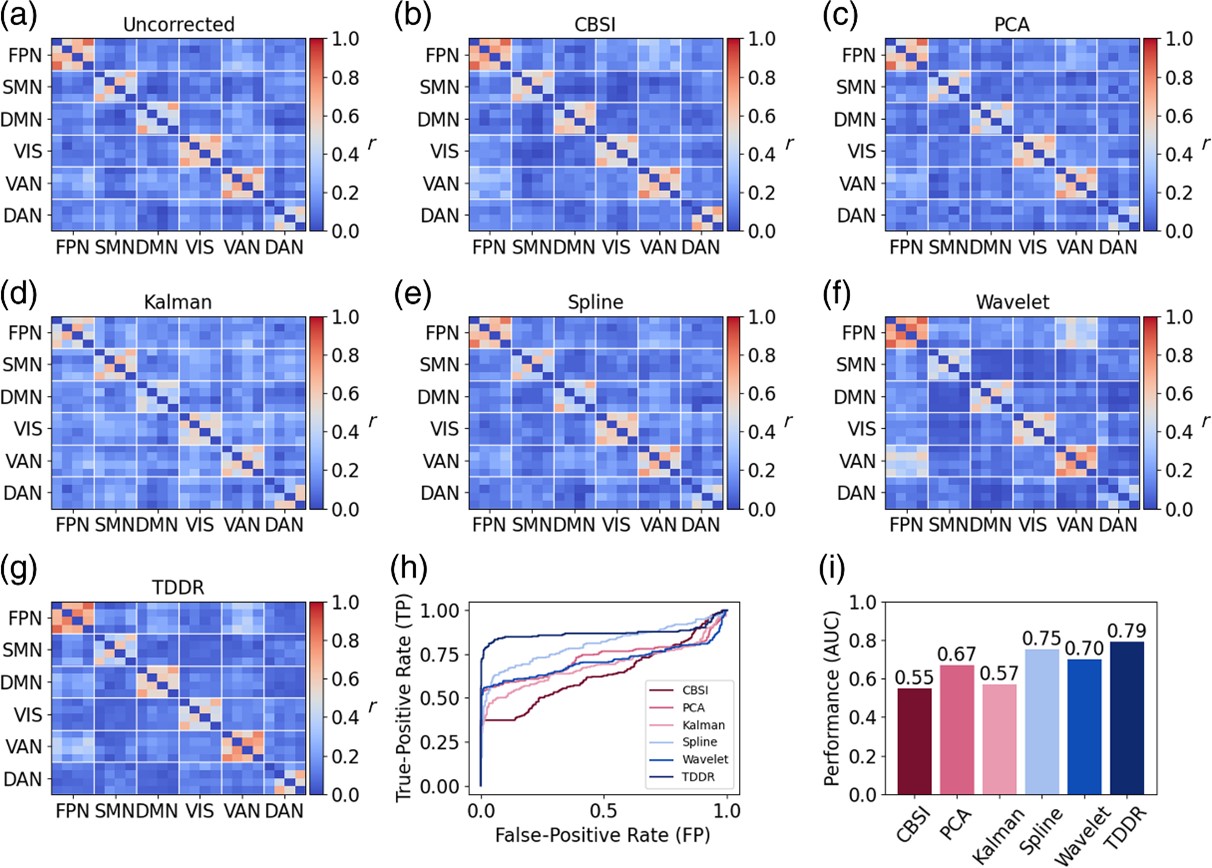
Fig.2. Visualization of the uncorrected and corrected functional connectivity (r) of each network in the experimental data (a)-(g). (h) and (i) Comparison of ROC and AUC obtained from different methods.
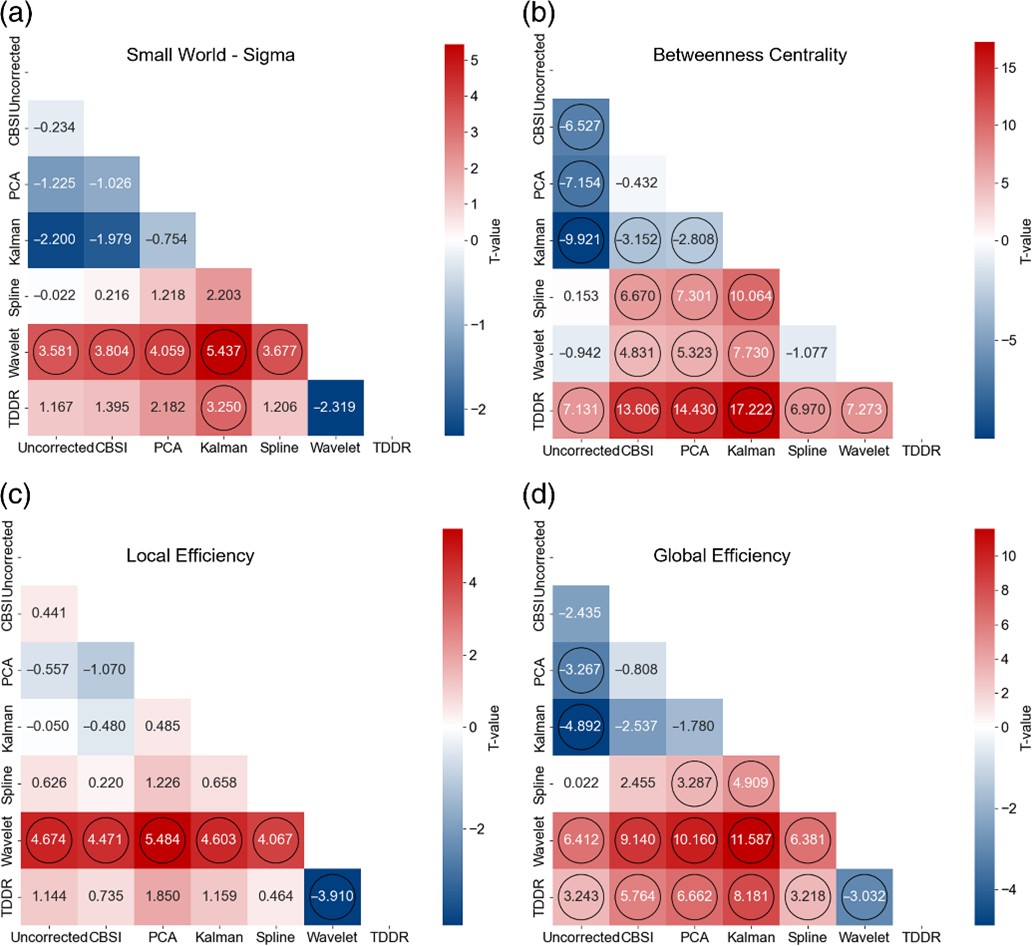
Fig.3. Statistical comparison of graph theory-based topological measures in the experimental data.