A collaborative team led by Prof. Yonghua Zhao from Institute of Chinese Medical Sciences and Prof. Zhen Yuan from the Center for Cognitive and Brain Sciences at the University of Macau, has developed a novel strategy to detect neurocognitive disorders (NCDs) associated with cognitive aging, by fusing electroencephalography (EEG) data and multi-omics profiles of the gut microbiome, metabolites, and urine exosomes. Their study was published in the reputable and influential international journal Microbiome (Five-Year Impact Factor IF=19.4, JCR Q1) on January, 2024., demonstrating that this approach can achieve 92.69% accuracy in distinguishing NCDs patients from normal aging seniors, and also provides insights into the underlying mechanisms of NCDs.
NCDs are a group of conditions that impair cognitive functions and affect the quality of daily life. They are becoming a major public health concern due to the aging population and the lack of precise diagnostic methods and specific biomarkers. Current diagnostic methods, such as neuropsychological tests, brain imaging, and laboratory tests, are often subjective, invasive, expensive, or time-consuming.
The research team proposed a personalized diagnostic strategy for NCDs based on the fusion of EEG data and multi-omics profiles. They collected multimodal EEG data and fecal and urine samples from 50 seniors in Macau, including 29 NCDs patients and 21 normal aging controls, and performed metagenomics, proteomics, and metabolomics analysis to identify the multi-omics signatures and metabolic pathways associated with NCDs. They also verified their findings in aged mice using fecal microbiota transplantation (FMT).
It suggested that NCDs patients had lower EEG power spectral density in the alpha and beta frequency bands, especially in the frontal, temporal, and posterior regions of the brain, indicating reduced cognitive functions. Specific gut bacteria, such as Ruminococcus gnavus, Enterocloster bolteae, and Lachnoclostridium sp. YL 32, and metabolites, such as L-tryptophan, L-glutamic acid, gamma-aminobutyric acid (GABA), and fatty acid esters of hydroxy fatty acids (FAHFAs) were determined, which were significantly altered in NCDs patients compared to normal aging controls. These multi-omics signatures were related to the biosynthesis of aromatic amino acids and the tricarboxylic acid (TCA) cycle, which are important for neurotransmitter synthesis and energy metabolism in the brain. The researchers also confirmed that FMT from NCDs patients to aged mice induced similar changes in multi-omics profiles, suggesting a causality between the gut microbiome and NCDs.
Finally, the researchers used a support vector machine (SVM) algorithm, a type of machine learning technique, to construct a classification model based on the fusion of EEG data and multi-omics profiles. The model achieved 92.69% accuracy in classifying NCDs patients from normal aging controls, outperforming the models based on EEG data or multi-omics profiles alone.
The study highlights the potential of multi-omics profiling and EEG data fusion in personalized diagnosis of NCDs, with the potential to improve diagnostic precision and provide insights into the underlying mechanisms of NCDs. It also suggests that the approach is able to be extended to other neurological disorders, such as Parkinson’s disease and depression, and are integrated with other modalities, such as brain imaging and genetic data, to further enhance the diagnostic accuracy.
This work was jointly supported by Science and Technology Development Fund, Macau SAR (File nos. 0106/2019/A2, 0045/2021/A1, SKL-QRCM(UM)-2023–2025). The full version of the article can be viewed at: https://doi.org/10.1186/s40168-023-01717-5.
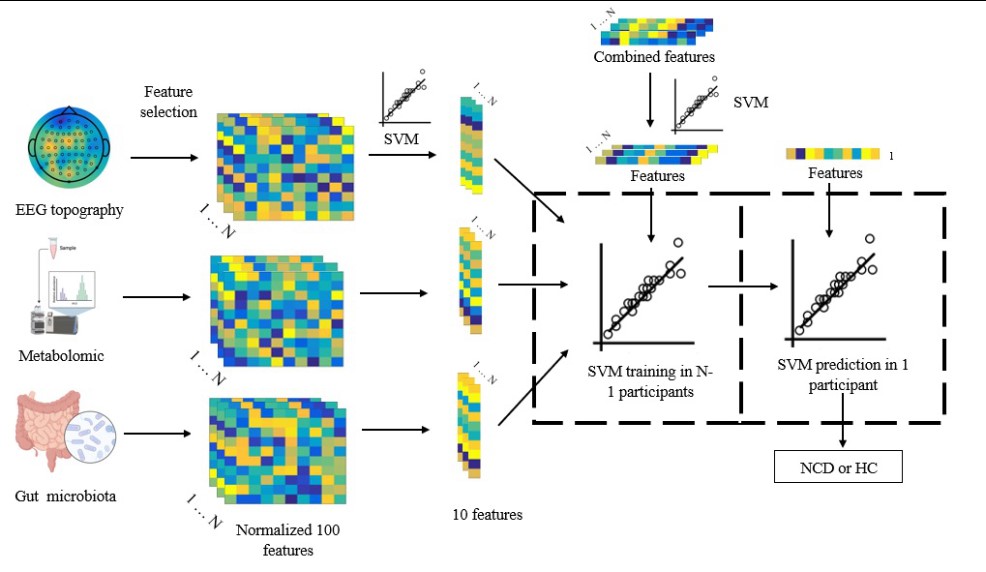
The fusion of multi-omics profile and multimodal EEG data
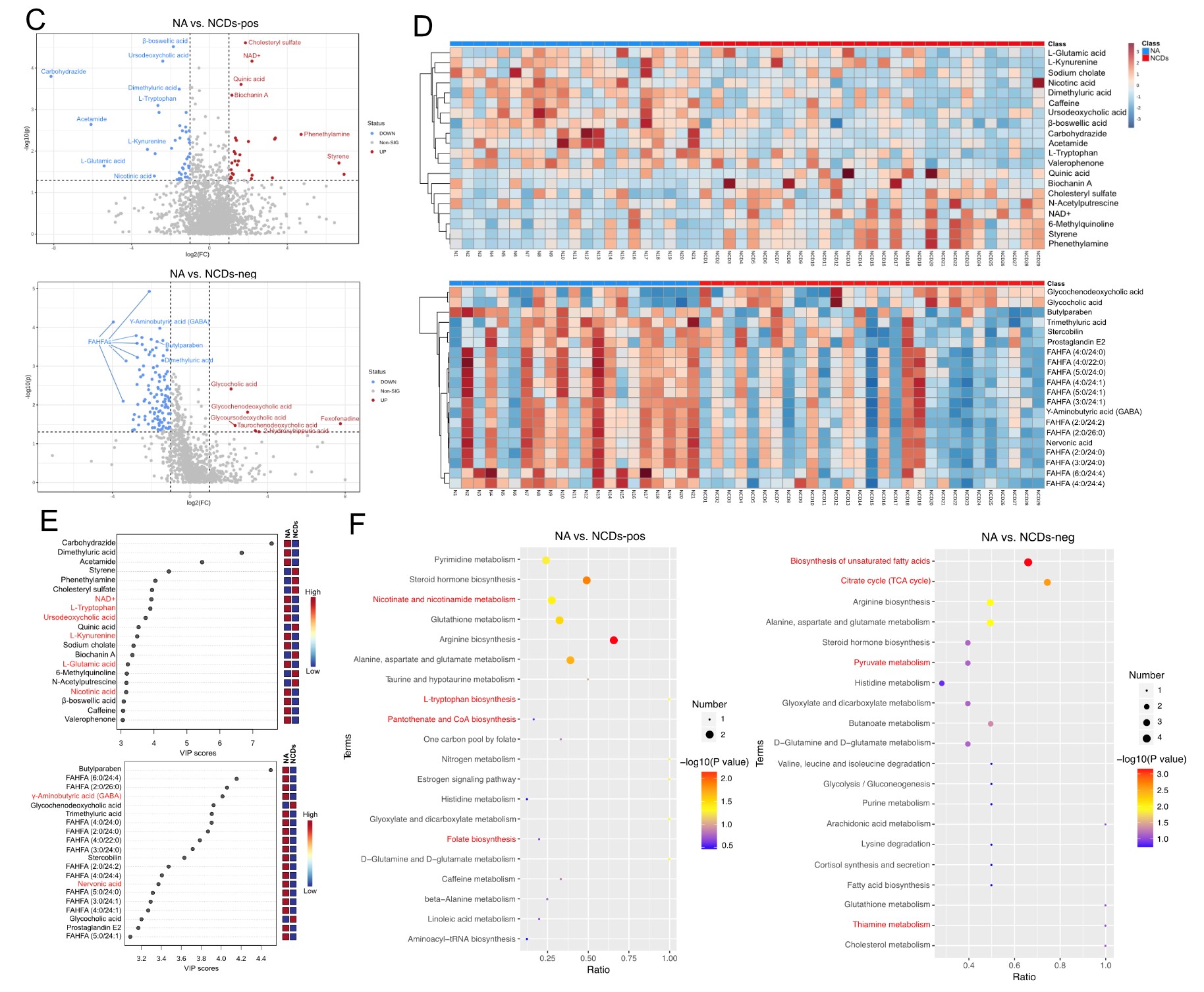
Intestinal metabolomic profiles of NCDs seniors
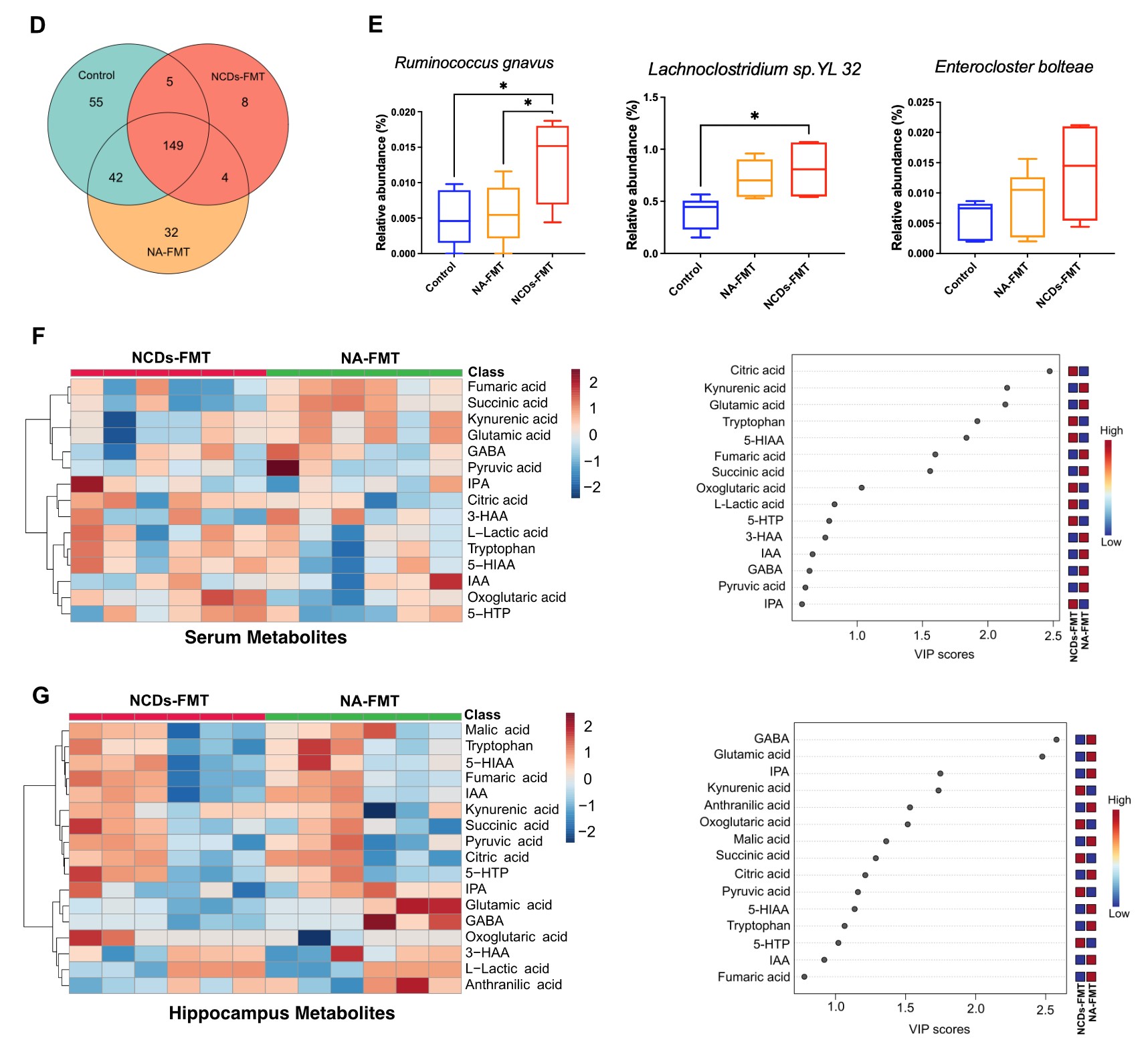
Intestinal, serum and hippocampal metabolomic profiles of FMT mice
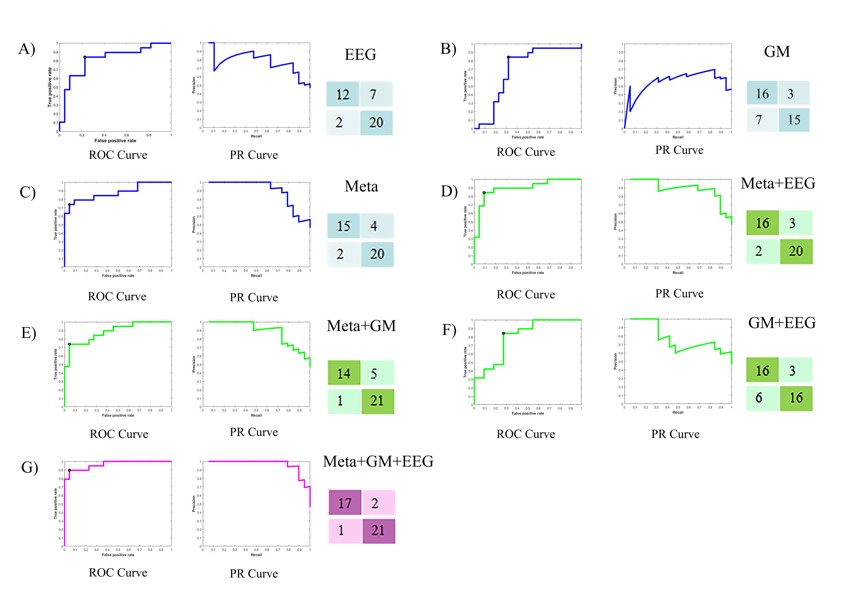
The machine learning performance of different omics