A team led by Yuan Zhen, professor at the Centre for Cognitive and Brain Sciences (CCBS) of the University of Macau (UM), has made new progress in the study of individual-specific functional connectivity to predict normal cognitive ageing (CA), mild cognitive impairment (MCI), and Alzheimer’s disease (AD). This study is the first to combine individual-specific functional connectivity with artificial intelligence (AI) techniques to reveal differences in functional connectivity between CA, MCI, and AD in elderly people with different APOE ε4 genotypes. The research results have been published in Communications Biology, a journal from Nature.
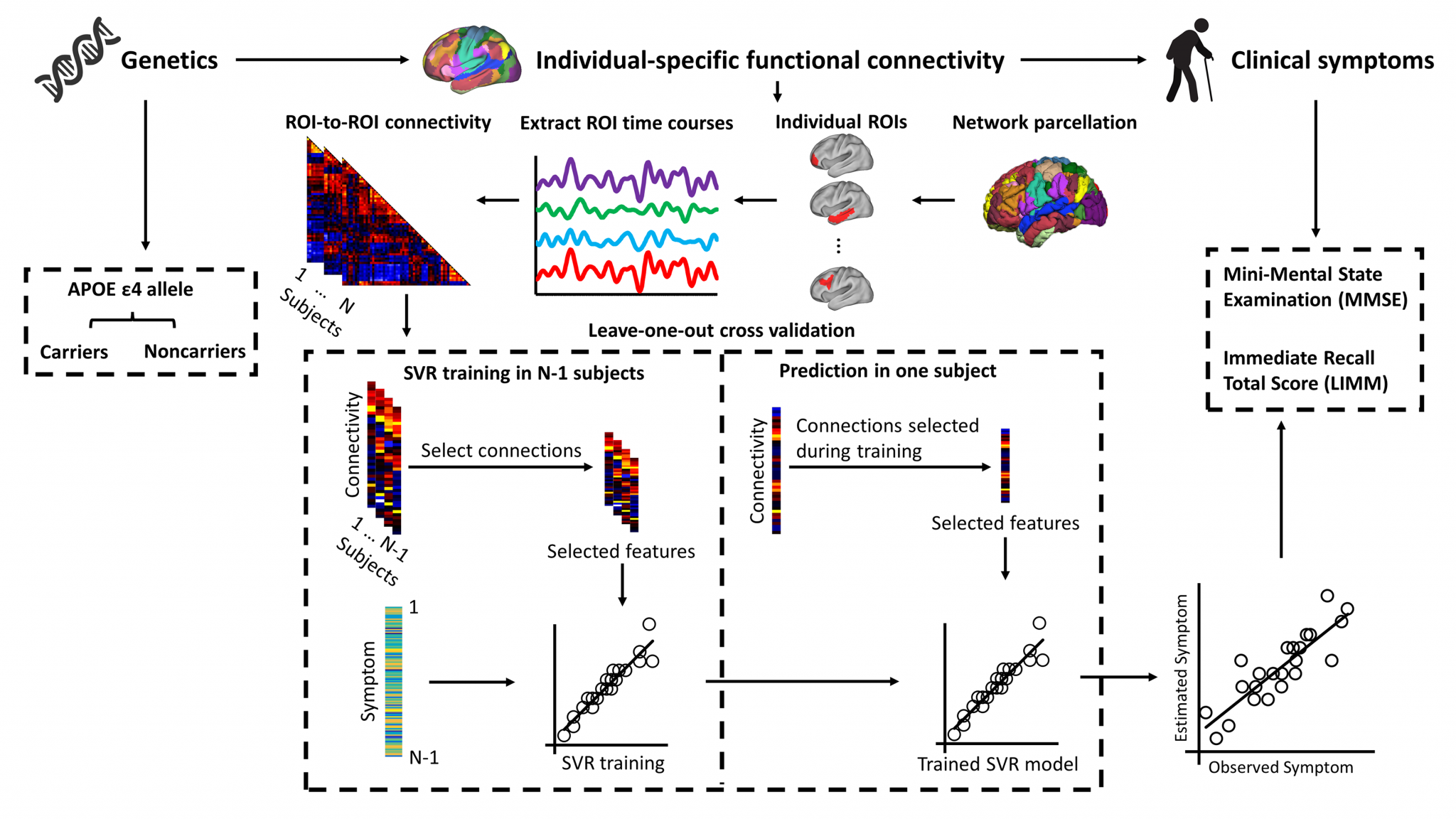
Figure 1. Clinical symptoms related to AD predicted by individual-specific functional connectivity in elderly populations with APOE ε4 carriers and non-carriers.
AD is one of the most common neurodegenerative diseases. As of 2019, over 5.16 million people worldwide were diagnosed with AD or other types of dementia. AD patients usually suffer from significant memory loss and executive function impairments, which lead to decreased quality of life. Traditional neuroimaging investigations relying on population-level functional network atlas have difficulty in identifying brain biomarkers for the accurate diagnosis of AD. At the individual level, studies on predicting the process from CA, MCI, to AD are still insufficient, particularly in patients presenting different clinical phenotypes.
In the study, researchers extracted the neuroimaging data of 235 elderly individuals (120 APOE ε4 carriers and 115 APOE ε4 non-carriers; age: 60-85 years) from the Alzheimer’s Disease Neuroimaging Initiative (ADNI) dataset and constructed individual-specific functional connectivity for each participant. They then utilised recursive feature selection-based machine learning to reveal individual brain-behaviour relationships and predict changes in the clinical symptoms in AD patients with different genotypes. The study found that individual-specific functional connectivity of elderly people with different APOE ε4 genotypes could be used to predict the clinical subgroups from CA, MCI, to AD, and yield moderate-to-strong estimation levels of AD-related clinical symptoms. However, the team failed to detect clinical symptoms in almost all cases with conventional atlas-based functional connectivity.
The study also showed that connections between brain functional networks are more informative than those within the same network in explaining symptom-specific severity of AD, particularly for elderly people with the APOE ε4 allele. The research results highlighted the importance of accounting for individual variation in cortical functional anatomy in neurodegenerative research, which can be extended to the healthy population. In addition, individual-specific functional connectivity models could be used to tailor treatment to specific needs. Thus, the study underlined the relationship between individual-specific functional connectivity and clinical symptoms, which can provide clinical utility for the diagnosis, prognosis, and treatment of AD.
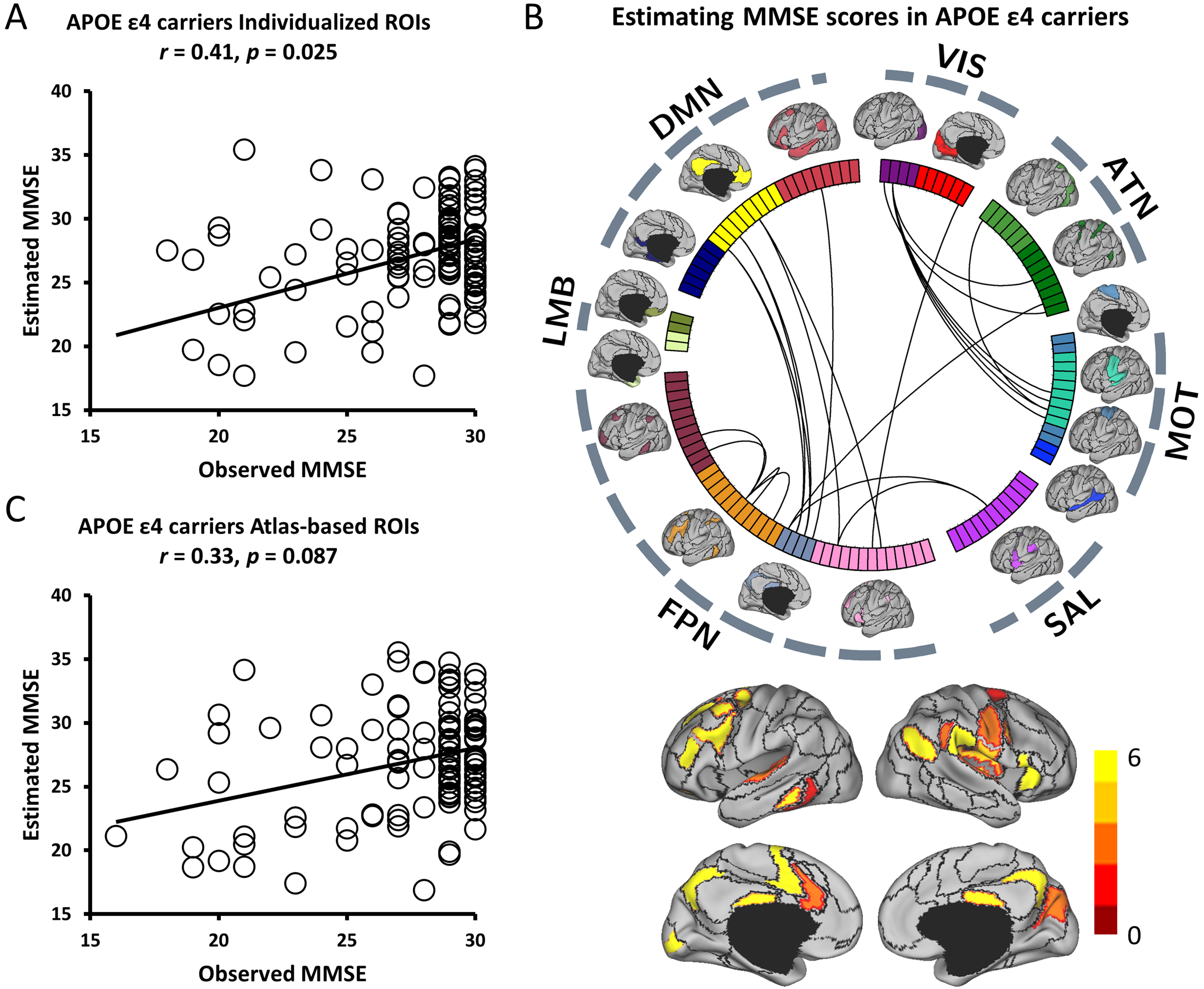
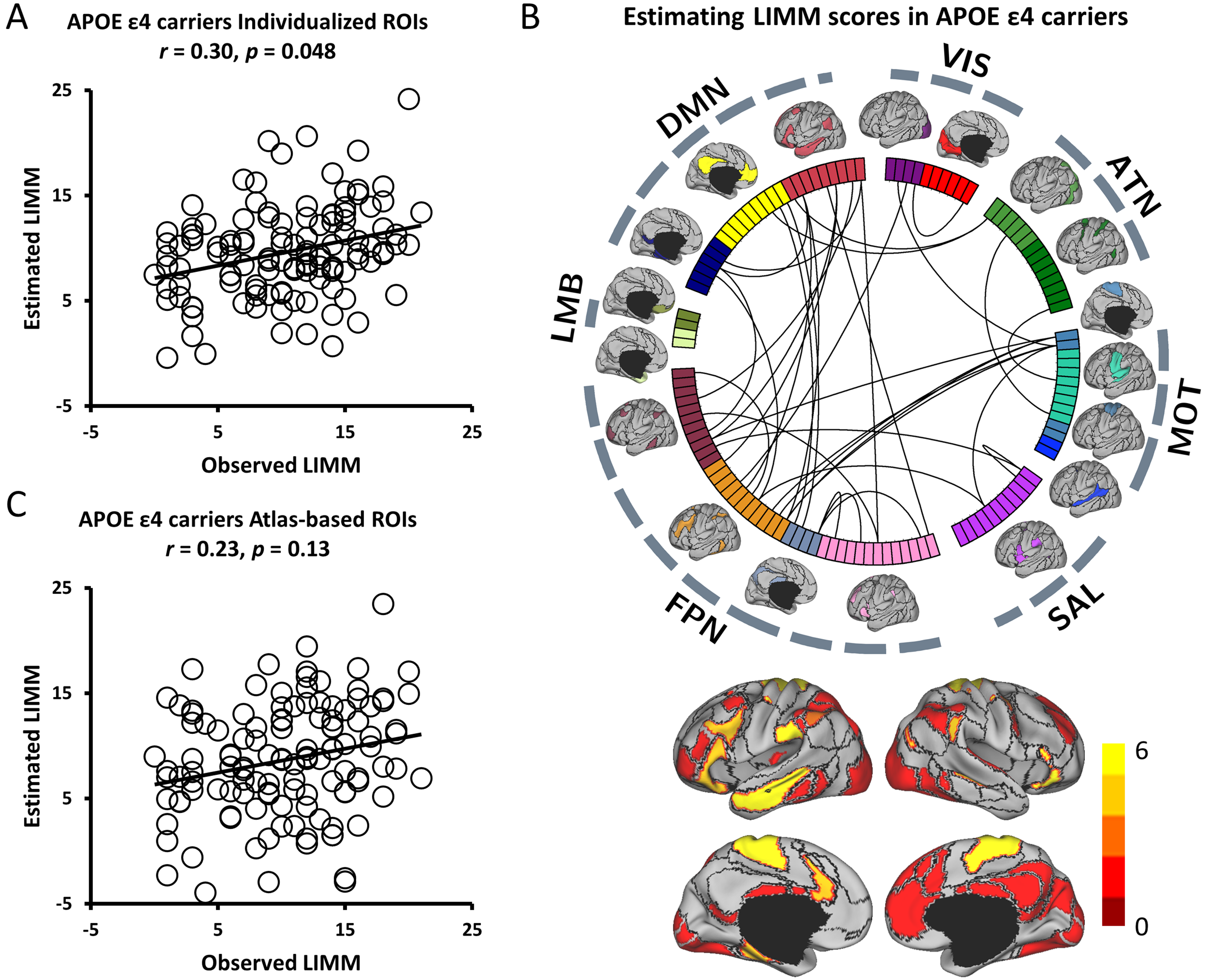
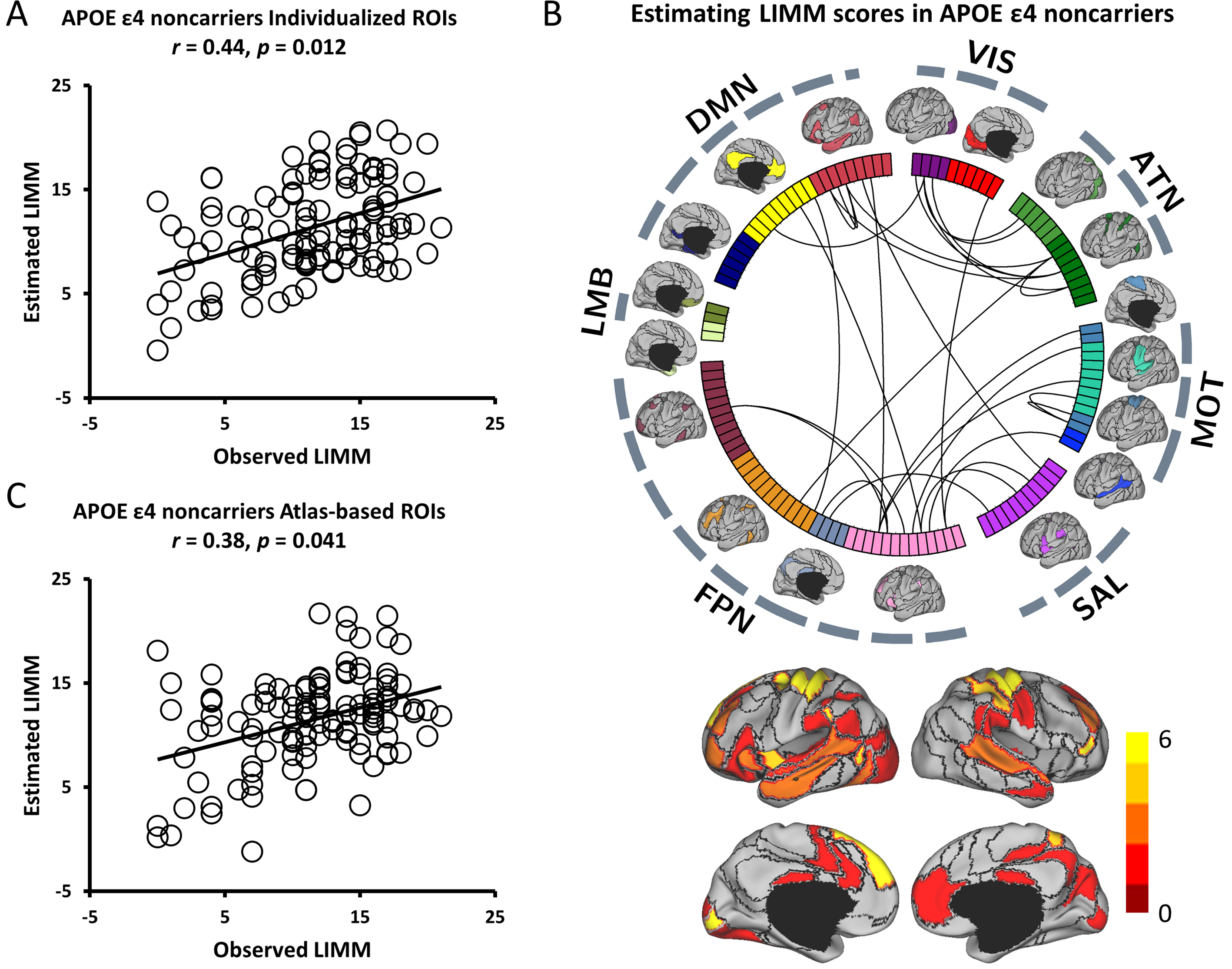
Fig. 2 Individual-specific functional connectivity can better predict AD symptoms as compared to traditional atlas-based functional connectivity.
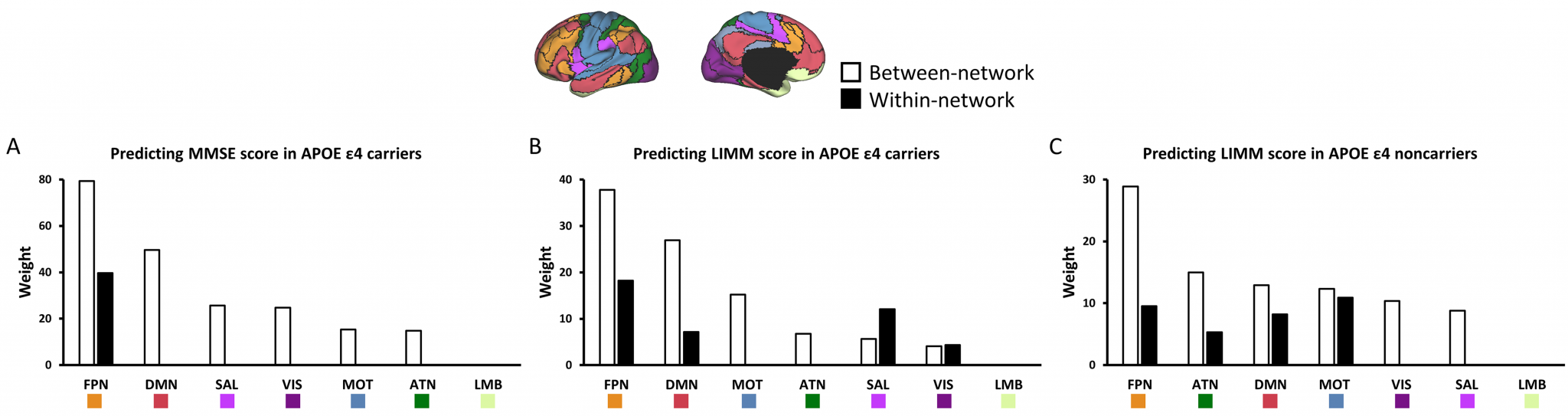
Fig. 3 Connections between individualized brain functional regions play an important role in predicting AD symptoms.
The study was led by Prof Yuan, and the first author of the paper is Hua Lin, PhD student in the CCBS and the Faculty of Health Sciences. The study was supported by the Science and Technology Development Fund, Macao SAR (File no: 0048/2021/AGJ, 0020/2019/AMJ), Macao SAR Government Education Fund (CP-UMAC-2020-01), and UM (MYRG2020-00067-FHS, MYRG2019-00082-FHS). The full version of the paper can be viewed at https://www.nature.com/articles/s42003-023-04952-6.
Paper Information:
Hua, L., Gao, F., Xia, X. et al. Individual-specific functional connectivity improves prediction of Alzheimer’s disease’s symptoms in elderly people regardless of APOE ε4 genotype. Commun Biol 6, 581 (2023). https://doi.org/10.1038/s42003-023-04952-6
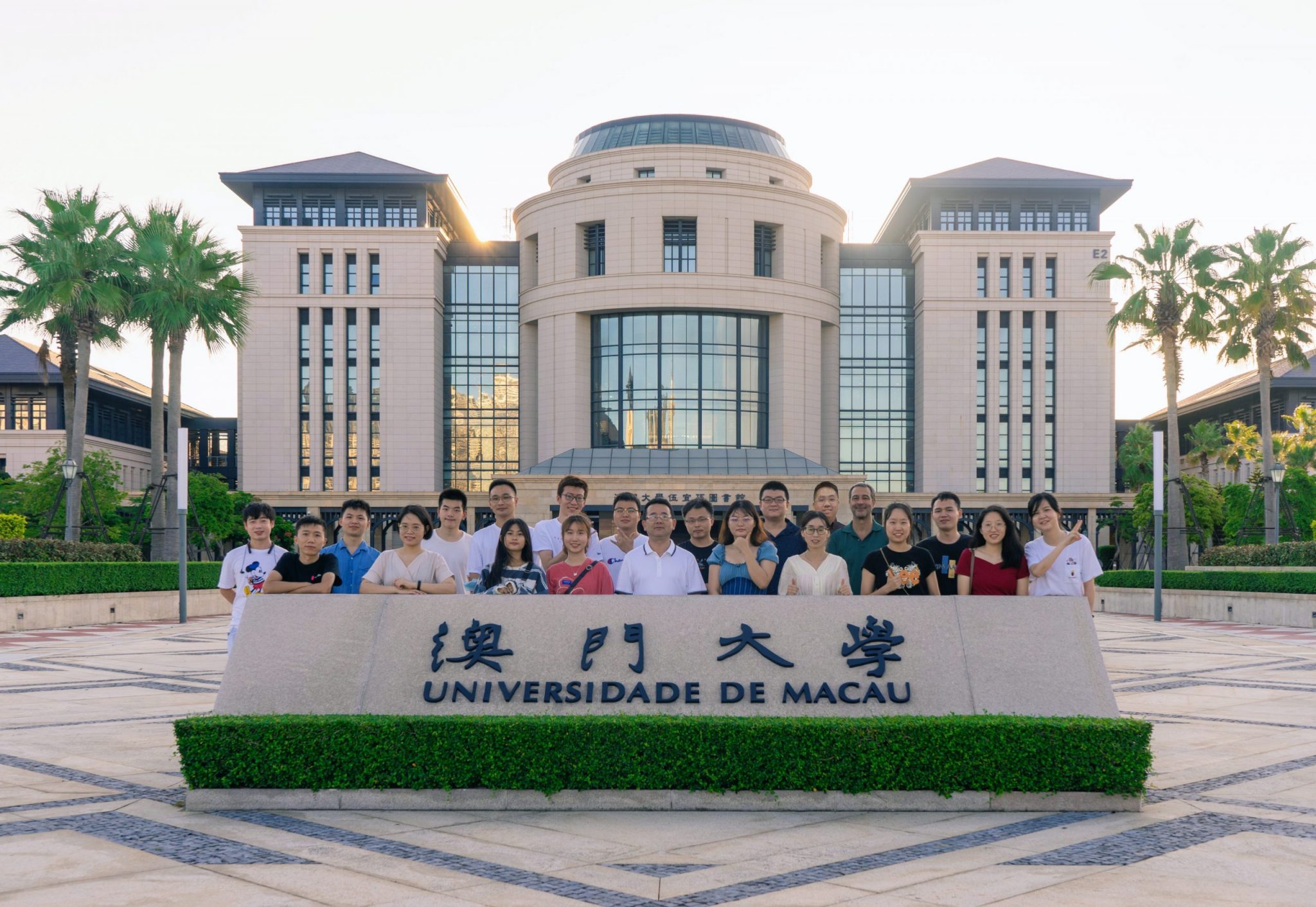
Yuan Zhen (middle) and Hua Lin (5th from left, 2nd row)